XENON and NVIDIA DGX Systems The Agile Platform for AI
21 Mar 2022
Graphics Processing Units have transformed data science
NVIDIA DGX Systems drive agile AI tackle the world’s most complex problems and reshape the future of learning with artificial intelligence.
Executive Summary
Artificial intelligence has moved from the pages of science textbooks to every corner of modern society. Higher education and research institutions are perfectly placed to lead the way, from grassroots innovation to applying AI across every aspect of our lives. From developing a new vaccine at previously unimaginable speed to creating greener materials for construction, discovering lower-impact energy sources and building autonomous machines, today’s research landscape demands hands-on AI skills. Entrusted to build the next generation workforce, universities must offer the AI-skills necessary to meet the demands of the future.
Are You Ready for the Big Bang of Modern AI?
In an accelerating world, it’s hard to think of any technology that’s speeding up faster than AI. Artificial intelligence, machine learning (ML) and deep learning are transforming every part of our lives, turning big data into discovery at blinding speeds and with more accuracy than the human mind could ever achieve.
World-leading high-performance computing is essential to support the AI revolution across universities and research institutions. NVIDIA has invested billions of dollars in creating the NVIDIA DGX™ A100, a universal system designed for all AI workloads, which is available as a fully integrated system offering unparalleled power, scalability and flexibility.
Around the world, top universities and research hospitals and institutions are advancing their work using NVIDIA DGX Systems (so, too, are numerous industries, including telcos, banks, manufacturers and automobile and aerospace companies).
AI researchers have precious expertise. Their work spans all disciplines and delivers the full spectrum of outcomes, from solving some of the world’s greatest problems to developing innovations that can cure, explain, preserve or simply delight, some of which we’ve not yet even dreamt. It’s imperative that the right instruments are available to support the brilliant minds of researchers and data scientists at work, which is why NVIDIA DGX Systems are such a powerful choice.
Developed specifically for AI workloads, the NVIDIA DGX A100 is the world’s first 5 petaFLOPS AI system and features the world’s most advanced accelerator, the NVIDIA A100 Tensor Core GPU. This system enables the consolidation of training, inference and analytics in a unified and easy-to-deploy AI infrastructure, supported by NVIDIA Enterprise Services, as well as XENON’s own local team.
Data analytics, AI and ML are already central to study and research work across all disciplines – from computational fluid dynamics in engineering to regression analysis in economics and everything in between. A NVIDIA DGX System is both a visionary and pragmatic investment for academic and research institutes as it provides the flexibility with easy processing configuration changes using the Multi-Instance GPU (MIG) feature.
Ramp Up With Containerisation
Institutions are unique and so are their compute requirements. NVIDIA DGX Systems are designed to scale up and scale out, enabling the computing system to stay in step with the cold reality of funding, as the work it’s performing explores and expands horizons.
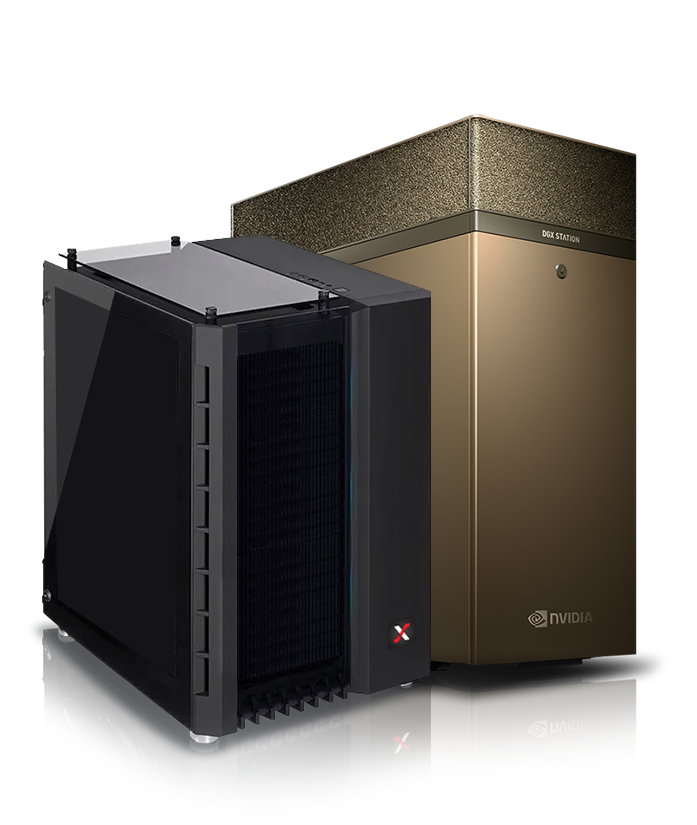
NVIDIA DGX Systems are serious investments. Universities and research institutes wanting to fast-track making high-end resources available could look at options such as partnering with each other to pool resources, build computing clusters based on NVIDIA DGX systems and perhaps share a NVIDIA DGX SuperPOD™, a proven architecture for DGX clusters enabling multi-tenant shared access.
For higher education and research, scalability is invaluable. Researchers and students save hours of time using the NVIDIA® NGC™ catalog of software containers and pretrained models optimized to run on DGX Systems. This facilitates starting small, and growing quickly and easily as the research grows.
A starting point for researchers is to begin with a single GPU workstation, such as the DGX Station™ or XENON DevCube, and use it to test models to show the promise of a theory, using early success to obtain funding to step up their compute power. These GPU workstations provide a data centre at the desk, with incredible processing power available with standard power and ambient cooling making them ideal for individuals and small teams. As theories are proven and compute requirements grow requiring larger data sets and more processing to take the research to the next level, the containerisation of the NVIDIA software stack makes it simple to scale up into the next DGX System. The same base operating system and quality-assurance testing between these systems ensure easy and predictable interoperability. The NVIDIA models operate in standard containers, allow them to be scaled seamlessly to larger DGX Systems. DGX A100 or a cluster of DGX Systems configured in a POD or SuperPOD allows the ultimate in scale.
This unique NVIDIA architecture is standardised on the widely used Linux operating system with NVIDIA drivers, which enable the functionality of the GPUs, making it possible to deploy the same software stack across all these solutions.
Scale Up And Out With Truly Agile Infrastructure
Unlike a lot of systems, the modularity of the DGX is not limited in one direction. As well as scaling out with more DGX systems, you can scale up by using Mult-Instance GPU (MIG) to combine GPU instances for a single job, increasing processing power effectively on-demand. When not combined into a single massive GPU, the GPU instances can also be allocated to individual researchers. This agility to divide the workload among other requirements, whether that’s several jobs within one project, different experiments in one research institute, or multiple faculties across one or more universities has fundamentally changed the previously fixed notions of infrastructure and created an agile, flexible platform for innovation.
Multi-Instance GPU (MIG) capability makes it simple to share the resource across faculties or even different universities or research institutes. The NVIDIA A100 Tensor Core GPUs – there are eight of them inside a NVIDIA DGX A100 – can each be sliced into seven smaller logical GPUs instances – each with dedicated high-bandwidth memory, cache and compute cores. The beauty of this is that it makes it possible to assign the GPU capacity to multiple users using the same machine at the same time or combine all 56 instances into a massive, powerful GPU. For example, the seven MIG instances could be used for inference processing during the day, and combined at night into a single instance GPU for massive deep learning processing. The right-sized GPU capacity can be assigned for any AI workload, with guaranteed quality of service, ensuring full optimisation of valuable resources and extending the reach of accelerated computing to all users. The availability of such precise allocation means that this valuable resource should never be sitting idle.
With MIG, multiple GPU instances can power AI workloads, right-sized for the needs of students and researchers or as a dual use platform for teaching and research. As compute requirements change over time, the ability to combine and isolate the MIG instances allows for the ultimate in flexibility.
XENON’s higher-education specialists work with university and research institutes to understand specific requirements and develop a plan to make it work today and into the future, knowing that the NVIDIA DGX is architected to deliver scalability, modularity and shareability.
How Harrison.ai Used NVIDIA DGX To Help Make Babies
The test, learn and scale approach is exemplified beautifully by Harrison.ai, a Sydney-based company founded by using AI and deep learning to develop and commercialise world-leading healthcare products and services.
A notable success has been the application of AI to improve IVF outcomes, working with Virtus Health, an Australian IVF provider, to develop a platform known as Ivy.
The emotional pain of failed embryos has been felt by many couples who’ve had to embark on the IVF journey. Harrison.ai has successfully refined the IVF process, using computer vision AI models, to vastly improve outcomes after embryos are created.
Photos of IVF embryos are taken every 10 minutes as the cells divide over the first five days, then compiled into a video. The computer vision AI model is applied to the time-lapse video and, continuously building on datasets, delivers increasingly accurate predictions of which embryos are most likely to result in viable pregnancies.
Humans are still very much in the equation – embryologists make the final decision on which embryos are selected for transfer, but the combination of detailed data sets and AI/ML technologies enables them to make better decisions, faster. Ivy perpetually self-learns, meaning accuracy and predictability continue to improve.
XENON worked with Harrison.ai from the very beginning, when models were first run in a workstation form factor, using an NVIDIA DGX Station. As results from the proof of concept grew stronger, the model evolved and as data expanded to many petabytes it was time to upgrade. XENON worked with the team to upgrade to NVIDIA DGX A100 Systems and high-speed storage. As the company continues to grow, it continues to build its capability by adding more DGX Systems into their growing cluster.
NVIDIA NGC catalog was essential, enabling Harrison.ai to go from the workstation environment to the DGX Systems as their compute requirements grew – it was simply a matter of taking the containers and the dataset across to the NVIDIA DGX System. Harrison.ai has since developed a radiology diagnostic tool, Annalise CXR using the same infrastructure and approach.
As Harrison.ai co-founder Dr Aengus Tran says, “What we aim to do is to harvest the power of AI and deep learning, and taking advantage of GPU-accelerated computing to provide healthcare at a cheaper cost, faster and at a higher quality, a triad that traditionally never really happened. My dream for AI is eventually we’ll get to a point where some of the difficult decisions that we need to make in healthcare will be largely automated by AI and free up to doctors to do what doctors are good at, which is empathising with the patient, catering the treatment to specific patients and their social circumstances, and develop affordable healthcare for the masses … I can hardly think of anything more stimulating to work on.”
Image supplied by Harrison.ai
AI Centres Of Excellence In Higher Education And Research
“With GPUs in supercomputers, we gave scientists a time machine,” NVIDIA founder and CEO Jensen Huang said in his keynote for GTC 21. “A scientist once told me that because of NVIDIA’s work , he could do his life’s work in his lifetime. I can’t think of a greater purpose.”
It sums up why AI must be a university-wide, cross-domain vision and why every university must be ready to offer AI capabilities to its students, faculty and researchers.
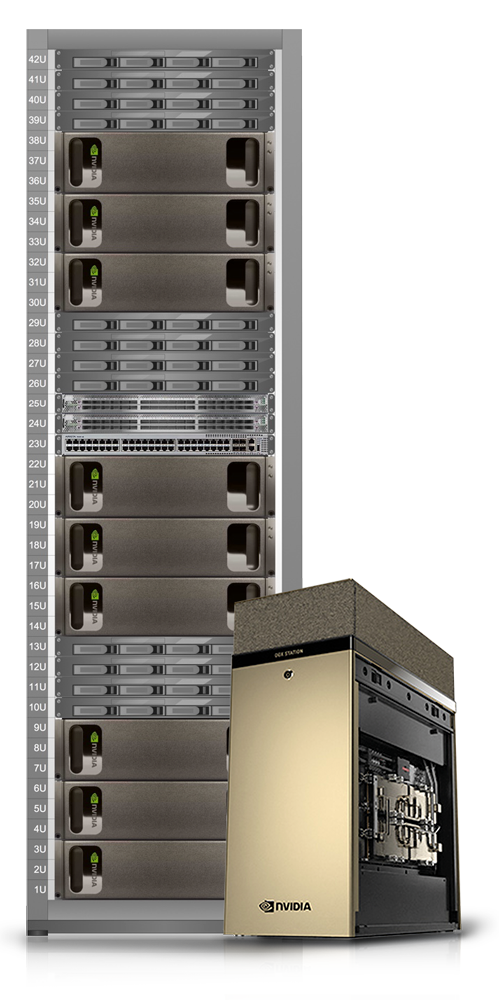
The NVIDIA DGX Station A100 makes it possible, bringing AI supercomputing performance to data-science teams and offering data-centre performance in a box without the need for a traditional data centre overheads of power and cooling. The system has four fully interconnected NVIDIA A100 Tensor Core GPUs either 160 or 320 GB of GPU memory and support for Multi-Instance GPU (MIG). It plugs into a standard wall outlet resulting in a powerful AI appliance which can be deployed almost anywhere. The plug and play nature of the DGX Station A100 starts delivering results within an hour of unboxing.
XENON regularly helps universities and research institutes plan and deploy the right NVIDIA DGX solution for their needs. The multi-tenant capabilities of MIG create a flexible pathway, opening up superfast GPU computing across a university for work that includes everything from data analytics and data science work, to AI model training, and AI inference processing.
Creative IT departments and faculty heads can collaborate on ways to fund clusters such as NVIDIA DGX POD and DGX SuperPOD and the high-performance storage required to ‘feed’ them efficiently. The DGX PODs and SuperPODs are pre-configured, proven architectures for clustering multiple DGX Systems into a unified high performance computing cluster. The designs include all switching, cabling, power and rack layouts – saving months of cluster design time.
Implementing DGX clusters create possibilities, including charge-out models or pooled resources to bring superior AI computing power to the institution, which in turn will create a positive feedback – and funding – loop. This high-powered NVIDIA DGX compute infrastructure will attract top graduate students to join the university, and because research cycles run so much faster and with more precision, researchers are able to move faster with their own work. This means they have a higher probability of success with grant applications, and that funding will help drive continuing investment in the system.
XENON regularly assists universities and research institutions to set up private cloud infrastructure in house, on-premise. The XENON private cloud for the Walter and Eliza Hall (WEHI) delivers on-demand computing resources and the experience of on-demand agility which can match IT infrastructure costs to project and grant budgets. The private cloud approach ensures efficient resource utilisation by making the infrastructure composable and available to more teams. Overall costs are much lower than public cloud at this scale, and WEHI also have the benefits of complete control of their data protection and data sovereignty.
What will your researchers be able to achieve when they have access to the fastest and best shared compute and data-storage resources? It’s time to find out.
XENON – Enabling Researchers to Do Great New Things
For over 25 years XENON has been delivering solutions for universities and researchers who are looking to iterate faster, collaborate more, and implement cutting edge research.
Explore the resources below, or get in touch with the XENON team to explore how NVIDIA DGX Systems can assist your team.
Get a QuotePOWER, SPEED, PRECISION
NVIDIA Multi-Instance GPU (MIG) in a single NVIDIA DGX A100 splits the 8 GPUs into 56 independent logical units which can be utilised singularly or in any combination.
Compared to a traditional CPU based data center for AI workloads, a NVIDIA DGX A100 data centre is about 1/10th of the cost, uses about 1/20th of the power and 1/25th of the space.
2.5 million developers and 7500 AI startups are creating thousands of applications for accelerated computing using NVIDIA DGX systems.
“Building tools for the Da Vincis of our time is our purpose, and in doing so we also help create the future.”
— NVIDIA Founder and CEO Jensen Huang